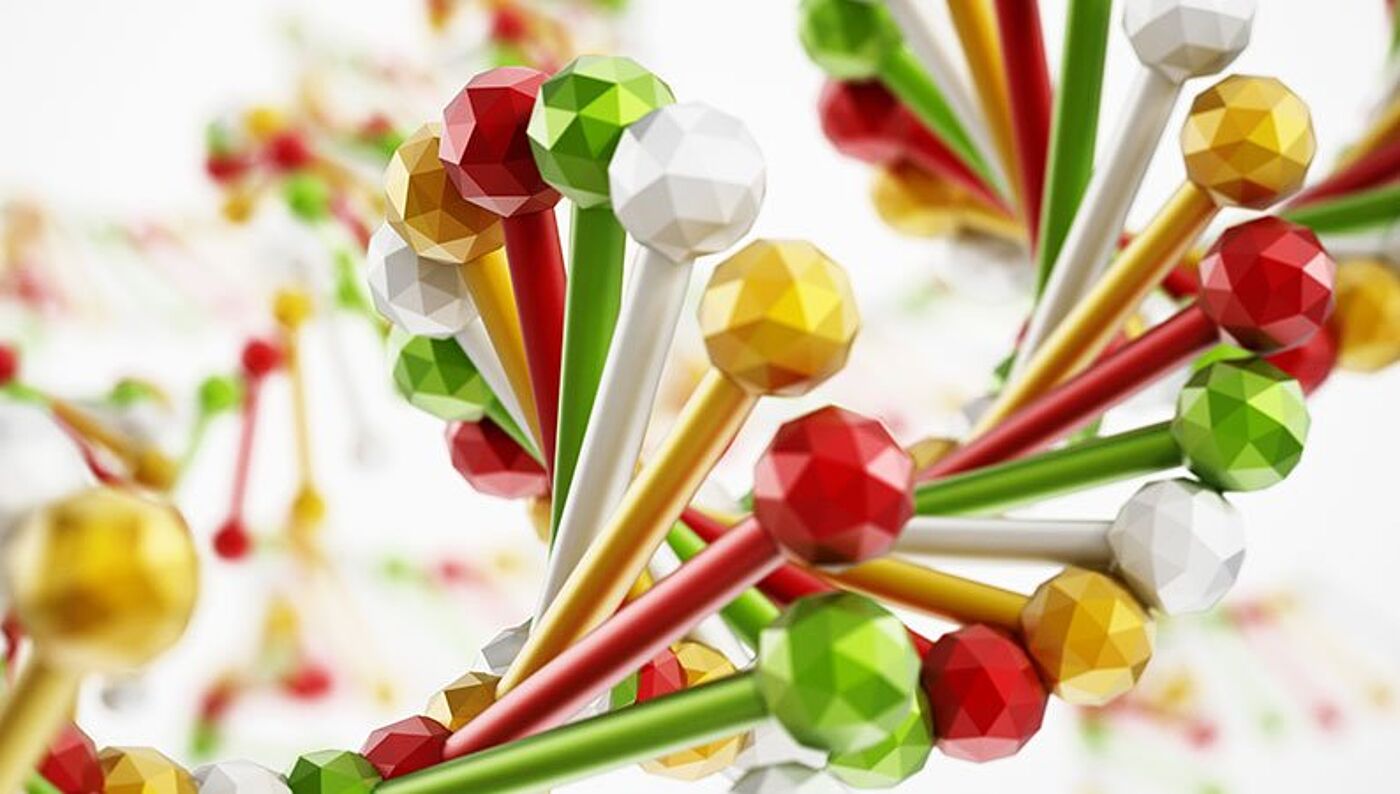
Decoded: DNA sequencing and patent similarity
Curiosity is the spark that drives all innovation and research. Nowadays, most of these endeavors result in documents such as patents that present newly achieved information and preserve it as the foundation for whatever might follow.
As all innovators will tell you, the trouble with any new project is finding the right information. But humans were not the first to work with information and data analysis. It was Mother Nature. Let us see how the quality of a patent search can be improved by solving essential questions about the essence of life forms – their DNA.
The big data of life
Nature uses an approach to storing and passing on information that is very different from ours. Like an instruction manual that guides about all sequential tasks in the right order to accomplish a process, biological organisms have all the details in their genes. They create combinations of nucleotides that produce the diversity we see in the biological world.
There are two types of nucleic acids: deoxyribonucleic acid (DNA) and ribonucleic acid (RNA). The sequence of DNA is of crucial importance as it contains the code to the formation of diverse proteins and hence the complexity and diversity of life. The unique order of bases in DNA results in the creation of basic hereditary units called genes. The process of decoding a DNA sequence into a protein is commonly referred to as the "central dogma of life."
One main challenge in sequence analysis is decoding the vast number and length of sequences. This big data of protein and DNA sequence databases (over 100 million sequences) comes from species across the tree of life. Sequence analysis can be done using conventional methods such as alignment algorithms, heuristic search methods or statistics-based methods. Although the local alignment methods based on dynamic programming are quite accurate and guaranteed to find an optimally scored alignment, they are slow and not practical for sequence alignments against databases with millions of sequences.
Nature and artificial intelligence
Biological data provides a perfect use case for machine learning and artificial intelligence algorithms. This is the reason that researchers in the field of bioinformatics and computational biology have used statistical analysis and inference since the very beginning. Logistic regressions, Support Vector Machines (SVM) and Random Forests have been used in numerous applications ranging from prediction of protein sequence or structural elements to the classification of proteins into different structural and functional classes. With the development of deep neural networks, we observe an increase in the use of the algorithms like Long Short Term Memory (LSTM) and Convolutional Neural Networks (CNN or ConvNet) to predict the different features and behavior of proteins, e.g., protein contact prediction and prediction of post-translational modifications.

Machine learning methods are broadly divided into two types: supervised and unsupervised learning. Based on the inherent features of the data, if it is not labeled and cannot be assigned to any type, then classification is done using unsupervised learning. For instance, the classification of proteins into different groups based on their sequence similarity to each other. K-means clustering and Markov clustering can be used in unsupervised classification. On the other hand, if the data is labeled into different sets, this information can be used to train the computer by showing its positive and negative examples. Once the training is complete, the accuracy of training can be tested by using similar data not used in the training data set. Any classification technique following training and testing procedures using labeled data is termed supervised machine learning. Examples for this type of learning include SVM, Random Forest and CNN.
Patents – the ever-growing DNA of human innovation
The underlying goal of these ML and AI applications is to detect and predict patterns. This, of course, is a purpose that is useful whenever we need to make sense of big data. In the IP industry, these models can be particularly valuable when trying to index and find patents. After all, sifting through prior art and literature can sometimes feel like decoding the hidden information of DNA sequences. And therefore, we use models in patent search and analytics that are similar to the ones used in bioinformatics. To yield the best results, we have to stay up to date with the cutting edge technologies emerging in the fields of AI and ML applications. Luckily, at Octimine, scientific curiosity is in our DNA.
For more information on computer-based biological sequence analysis, access chapter 4 of "Computational Biology," which was contributed by Usman Saeed, Researcher and Data Scientist at Dennemeyer Octimine. Published by Codon Publications, the book "draws together many of the latest cutting-edge developments in the field of Computational Biology" with each chapter highlighting the utility of specific technologies.
Filed in
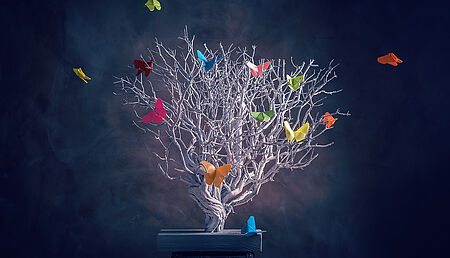
Learn how nine simple steps can help you overcome the challenges and rein in the costs of international patent filing.